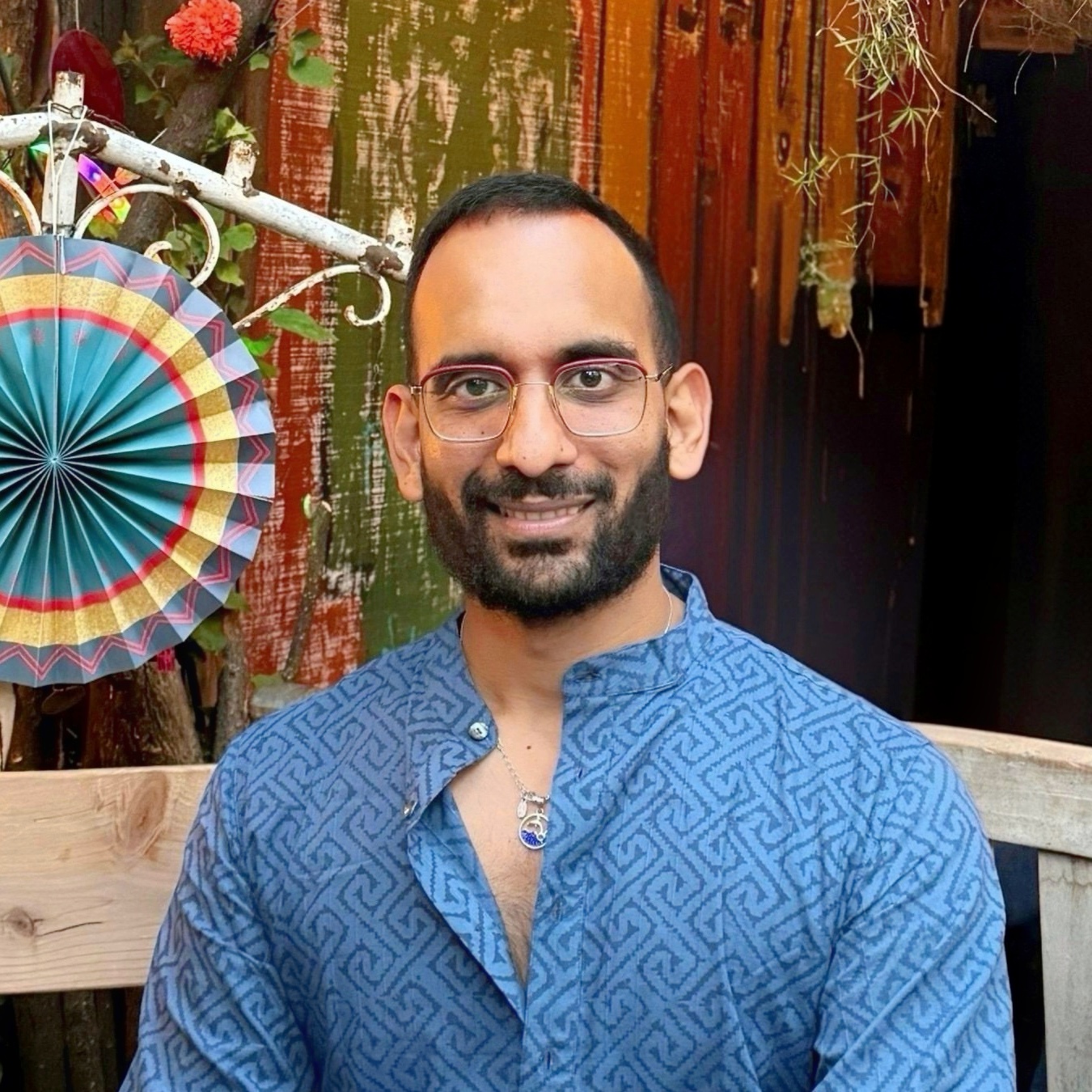
I am a Staff Research Scientist at Google Research in Mountain View, USA. I am broadly interested in the design of principled and practical algorithms for machine learning, and am currently focused on improving inference efficiency in large language models.
Prior to Google, I was a post-doctoral fellow at Harvard University (2015-2018), working with David C. Parkes. I completed my PhD in Computer Science from the Indian Institute of Science (2012-2015), working with Shivani Agarwal, and supported by a Google India PhD Fellowship. During my PhD, I also interned at Microsoft Research with Prateek Jain.
News & Highlights
-
2025
- ICLR 2025 Outstanding Paper Honorable Mention Award for our paper "Faster cascades via speculative decoding".
-
2024
- Serving as Senior Area Chair for NeurIPS 2024.
- Two journal papers accepted in JMLR and JACM.
- Three papers accepted at ICLR 2024, including a Spotlight presentation.
- New patent on "Dynamic selection from among multiple candidate generative models with differing computational efficiencies". [US20240311405A1]
- New patent on "Performing classification tasks using post-hoc estimators for expert deferral". [US20240135254A1]
-
2023
- Serving as Area Chair for ICML 2023.
- Invited talk on "Learning to abstain and beyond" at the 1st AAAI Workshop on Deployable AI (DAI). [slides]
-
2022
- New patent on "Systems and Methods for Implicit Rate-Constrained Optimization of Non-Decomposable Objectives". [US20220398506A1]
- Co-organized tutorial on "Deep AUC Maximization: From Algorithms to Practice" at CVPR 2022.
-
2021
- Serving as Area Chair for NeurIPS 2021.
-
2020
- Four papers accepted at NeurIPS 2020.
- One among top 10% reviewers in NeurIPS 2020.
- One among top 33% reviewers in ICML 2020.
- Guest lecture on "Fairness Goals through Constrained Optimization" in the AI and Ethics course at IIT Kharagpur.
- Co-authored Google blogpost on "Setting Fairness Goals with the TensorFlow Constrained Optimization Library".
-
2019
- Two papers accepted at NeurIPS for Oral Presentation (0.5% of submissions).
- One paper accepted at ICML for Oral Presentation (<5% of submissions).
- One among top 400 reviewers in NeurIPS 2019.
Research
The following are some themes I am excited about:
- Fast inference algorithms for large language models (LLMs).
- Design of loss functions for ranking, retrieval, and other real-world objectives.
- Generative LLMs for regression and ranking.
- Constrained optimization with application to fairness.
In the past, I have worked on applying machine learning techniques to the design of auctions and other economic mechanisms.
Selected Publications
Faster Cascades via Speculative Decoding.
In the 13th International Conference on Learning Representations (ICLR), 2025. [pdf]
Outstanding Paper Honorable Mention Award [details]
Wang, S., Guo, W.,
Robust Optimization for Fairness with Noisy Protected Groups.
In Advances in Neural Information Processing Systems (NeurIPS), 2020. [pdf] [details]
Feel free to also see NeurIPS 2021, ICLR 2022 (Spotlight), ICLR 2023, UAI 2023.
Cotter, A.,
On Making Stochastic Classifiers Deterministic.
In Advances in Neural Information Processing Systems (NeurIPS), 2019. [pdf]
Oral presentation [details]
Feel free to also see NeruIPS 2019b (Oral), NeurIPS 2020, AAAI 2020.
Duetting, P., Feng, Z.,
Optimal Auctions through Deep Learning.
In Proceedings of the 36th International Conference on Machine Learning (ICML), 2019. [pdf]
Oral Presentation
Longer version in the Journal of the ACM (JACM), 71(1): 1-53, 2024. [pdf] [details]
Culmination of work on machine learning for mechanism design (e.g., NeurIPS 2015, AAMAS 2018).
Learning with Complex Loss Functions and Constraints.
In Proceedings of the 21st International Conference on Artificial Intelligence and Statistics (AISTATS), 2018. [pdf]
Oral presentation
Longer version in the Journal of Machine Learning Research (JMLR), 25(367):1−81, 2024. [pdf] [details]
Feel free to also see NeurIPS 2014, ICML 2015, NeurIPS 2020.
Optimizing Non-decomposable Performance Measures: A Tale of Two Classes.
In Proceedings of the 32nd International Conference on Machine Learning (ICML), 2015. [pdf] [details]
Feel free to also see NeurIPS 2014, ICML 2015, KDD 2016, ICML 2021.
Majumder, B., Baraneedharan, U., Thiyagarajan, S., Radhakrishnan, P.,
Predicting Clinical Response to Anticancer Drugs Using an Ex Vivo Platform that Captures Tumour Heterogeneity.
Nature Communications, 6:6169, 2015.
[paper] [details]
A real-world application of the algorithms we developed for ranking with (partial) AUC in ICML 2013, KDD 2013, NeuralComp 2017.
Feel free to also see NeurIPS 2013 (Spotlight), ICML 2025.
Professional Service
Senior Area Chair: NeurIPS 2024, 2025
Area Chair: ICML 2023, 2024, 2025; NeurIPS 2021, 2022, 2023
Conference Reviewing: NeurIPS 2018 (top 200), 2019 (top 400), 2020 (top 10%); ICML 2020 (top 33%); ICLR 2021, 2022, 2023, 2024; FAccT 2021, 2022
Journal Reviewing: JMLR, TPAMI, TEAC, TMLR, JAIR, TKDE, Artificial Intelligence, Pattern Recognition Letters
Preprints
Jitkrittum, W.,
Universal Model Routing for Efficient LLM Inference.
Manuscript, 2025.
[arXiv:2502.08773]
Wang, C., Augenstein, S., Rush, K., Jitkrittum, W.,
Cascade-Aware Training of Language Models.
Manuscript, 2024.
[arXiv:2406.00060]
Cotter, A., Menon, A.K.,
Distilling Double Descent.
Manuscript, 2021.
[arXiv:2102.06849]
Publications
2025
Lukasik, M., Chen, L.,
Bipartite Ranking From Multiple Labels: On Loss Versus Label Aggregation.
In the 42nd International Conference on Machine Learning (ICML), 2025. To appear.
[arXiv:2504.11284]
Faster Cascades via Speculative Decoding.
In the 13th International Conference on Learning Representations (ICLR), 2025.
[pdf]
Outstanding Paper Honorable Mention Award
Lukasik, M., Meng, Z.,
Better Autoregressive Regression with LLMs.
In the 13th International Conference on Learning Representations (ICLR), 2025.
[pdf]
Spotlight presentation
2024
Consistent Multiclass Algorithms for Complex Metrics and Constraints.
Journal of Machine Learning Research (JMLR), 25(367):1−81, 2024.
Lukasik, M.,
Regression-aware Inference with LLMs.
In Findings of the Association for Computational Linguistics: EMNLP 2024.
Duetting, P., Feng, Z.,
Optimal Auctions through Deep Learning: Advances in Differentiable Economics.
Journal of the ACM (JACM), 71(1): 1-53, 2024.
Learning to Reject Meets Long-tail Learning.
In the 12th International Conference on Learning Representations (ICLR), 2024.
Spotlight presentation
Gupta, N.,
Language Model Cascades: Token-Level Uncertainty And Beyond.
In the 12th International Conference on Learning Representations (ICLR), 2024.
Post-hoc Estimators for Selective Classification and OOD Detection.
In the 12th International Conference on Learning Representations (ICLR), 2024.
2023
Jitkrittum, W., Gupta, N., Menon, A.K.,
When Does Confidence-Based Cascade Deferral Suffice?
In Advances in Neural Information Processing Systems (NeurIPS), 2023.
Wang, S., Narasimhan, N., Zhou, Y., Hooker, S., Lukasik, M., Menon, A.K.
Robust Distillation for Worst-class Performance.
In the 39th Conference on Uncertainty in Artificial Intelligence (UAI), 2023.
Wei, J.,
Distributionally Robust Post-hoc Classifiers under Prior Shifts.
In the 11th International Conference on Learning Representations (ICLR), 2023.
2022
Post-hoc Estimators for Learning to Defer to an Expert.
In Advances in Neural Information Processing Systems (NeurIPS), 2022.
Hiranandani, G., Mathur, J.,
Quadratic Metric Elicitation for Fairness and Beyond.
In the 38th Conference on Uncertainty in Artificial Intelligence (UAI), 2022.
Oral presentation
Jiang, H.,
Churn Reduction via Distillation.
In the 10th International Conference on Learning Representations (ICLR), 2022.
Spotlight presentation
2021
Training Over-parameterized Models with Non-decomposable Metrics.
In Advances in Neural Information Processing Systems (NeurIPS), 2021.
Hiranandani, G., Mathur, J.,
Optimizing Black-box Metrics with Iterative Example Weighting.
In the 38th International Conference on Machine Learning (ICML), 2021.
Kumar, A.,
Implicit Rate-constrained Optimization of Non-decomposable Objectives.
In the 38th International Conference on Machine Learning (ICML), 2021.
Duetting, P., Feng, Z.,
Optimal Auctions through Deep Learning.
Invited Research Highlight, Communications of the ACM (CACM), 64(8):109-116, 2021.
2020
Wang, S., Guo, W.,
Robust Optimization for Fairness with Noisy Protected Groups.
In Advances in Neural Information Processing Systems (NeurIPS), 2020.
Approximate Heavily-constrained Learning with Lagrange Multiplier Models.
In Advances in Neural Information Processing Systems (NeurIPS), 2020.
Hiranandani, G.,
Fair Performance Metric Elicitation.
In Advances in Neural Information Processing Systems (NeurIPS), 2020.
Tavker, S.K., Ramaswamy, H.G., and
Consistent Plug-in Classifiers for Complex Objectives and Constraints.
In Advances in Neural Information Processing Systems (NeurIPS), 2020.
Jiang, Q., Adigun, O.,
Optimizing Black-box Metrics with Adaptive Surrogates.
In Proceedings of the 37th International Conference on Machine Learning (ICML), 2020.
Pairwise Fairness for Ranking and Regression.
In Proceedings of the 34th AAAI Conference on Artificial Intelligence (AAAI), 2020.
2019
Optimizing Generalized Rate Metrics with Three Players.
In Advances in Neural Information Processing Systems (NeurIPS), 2019.
Oral presentation
Cotter, A.,
On Making Stochastic Classifiers Deterministic.
In Advances in Neural Information Processing Systems (NeurIPS), 2019.
Oral presentation
Zhao, S., Fard, M.M.,
Metric-optimized Example Weights.
In Proceedings of the 36th International Conference on Machine Learning (ICML), 2019.
Duetting, P., Feng, Z.,
Optimal Auctions through Deep Learning.
In Proceedings of the 36th International Conference on Machine Learning (ICML), 2019.
Oral presentation
Duetting, P., Feng, Z.,
Machine Learning for Optimal Economic Design.
The Future of Economic Design, Springer, 2019.
2018
Learning with Complex Loss Functions and Constraints.
In Proceedings of the 21st International Conference on Artificial Intelligence and Statistics (AISTATS), 2018.
Oral presentation
Golowich, N.,
Deep Learning for Multi-Facility Location Mechanism Design.
In Proceedings of the 27th International Joint Conference on Artificial Intelligence (IJCAI), 2018.
Feng, Z.,
Deep Learning for Revenue-Optimal Auctions with Budgets.
In Proceedings of the 17th International Conference on Autonomous Agents and Multiagent Systems (AAMAS), 2018.
2017
Support Vector Algorithms for Optimizing the Partial Area Under the ROC curve.
Neural Computation, 29(7):1919-1963, 2017.
2016
Optimizing the multiclass F-measure via biconcave programming.
In Proceedings of the 16th IEEE International Conference on Data Mining (ICDM), 2016.
Kar, P., Li, S.,
Online optimization methods for the quantification problem.
In Proceedings of the 22nd ACM SIGKDD International Conference on Knowledge, Discovery and Data Mining (KDD), 2016.
Automated Mechanism Design without Money via Machine Learning.
In Proceedings of the 25th International Joint Conference on Artificial Intelligence (IJCAI), 2016.
A General Statistical Framework for Designing Strategy-proof Assignment Mechanisms.
In Proceedings of the Conference on Uncertainty in Artificial Intelligence (UAI), 2016.
2015
Learnability of influence in networks.
In Advances in Neural Information Processing Systems (NIPS), 2015.
Ahmed, S.,
Bayes optimal feature selection for supervised learning with general performance measures.
In Proceedings of the 31st Conference on Uncertainty in Artificial Intelligence (UAI), 2015.
Majumder, B., Baraneedharan, U., Thiyagarajan, S., Radhakrishnan, P.,
Predicting clinical response to anticancer drugs using an ex vivo platform that captures tumour heterogeneity.
Nature Communications, 6:6169, 2015.
Consistent multiclass algorithms for complex performance measures.
In Proceedings of the 32nd International Conference on Machine Learning (ICML), 2015.
(*both authors contributed equally to the paper)
Optimizing non-decomposable performance measures: A tale of two classes.
In Proceedings of the 32nd International Conference on Machine Learning (ICML), 2015.
Kar, P.,
Surrogate functions for maximizing precision at the top.
In Proceedings of the 32nd International Conference on Machine Learning (ICML), 2015.
2014
On the statistical consistency of plug-in classifiers for non-decomposable performance measures.
In Advances in Neural Information Processing Systems (NIPS), 2014.
(*both authors contributed equally to the paper)
Kar, P.,
Online and stochastic gradient methods for non-decomposable loss functions.
In Advances in Neural Information Processing Systems (NIPS), 2014.
Saha, A., Dewangan, C.,
Learning score systems for patient mortality prediction in intensive care units via orthogonal matching pursuit.
In Proceedings of the 13th International Conference on Machine Learning and Applications (ICMLA), 2014.
Agarwal, A.,
GEV-canonical regression for accurate binary class probability estimation when one class is rare.
In Proceedings of the 31st International Conference on Machine Learning (ICML), 2014.
2013
On the relationship between binary classification, bipartite ranking, and binary class probability estimation.
In Advances in Neural Information Processing Systems (NIPS), 2013.
Spotlight presentation
SVM_pAUC^tight: A new support vector method for optimizing partial AUC based on a tight convex upper bound.
In Proceedings of the 19th ACM SIGKDD Conference on Knowledge, Discovery and Data Mining (KDD), 2013.
Menon, A. K.,
On the statistical consistency of algorithms for binary classification under class imbalance.
In Proceedings of the 30th International Conference on Machine Learning (ICML), 2013.
[paper]
[supplementary material]
A structural SVM based approach for optimizing partial AUC.
In Proceedings of the 30th International Conference on Machine Learning (ICML), 2013.
[paper]
[supplementary material]
Hobbies
Outside of work, I am passionate about baking, and particularly enjoy designing themed cakes (e.g., for Halloween and Pride). More recently, I have taken to amateur theatre. For more details about my personal hobbies, feel free to visit my Instagram page.
The following are some of my cake designs:
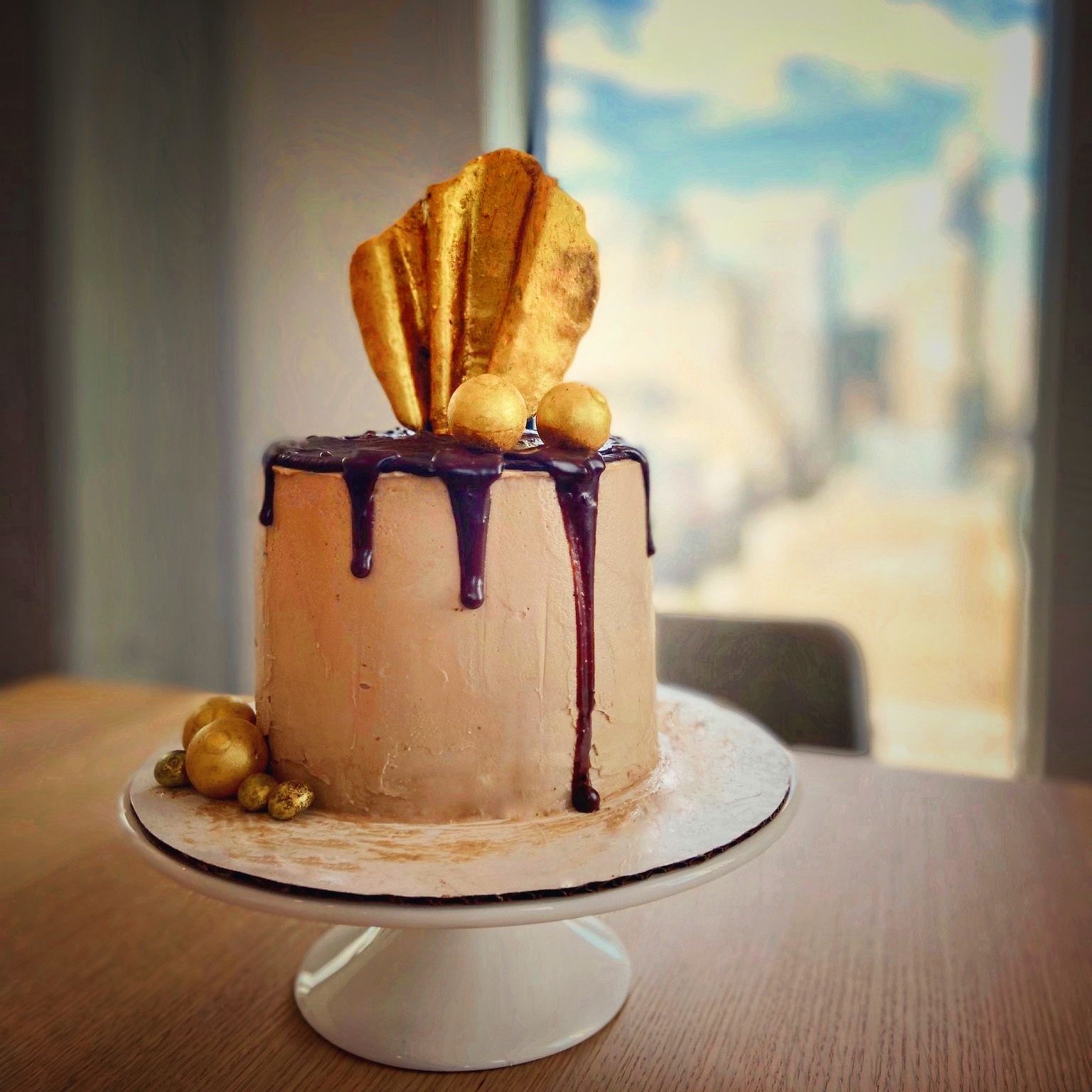
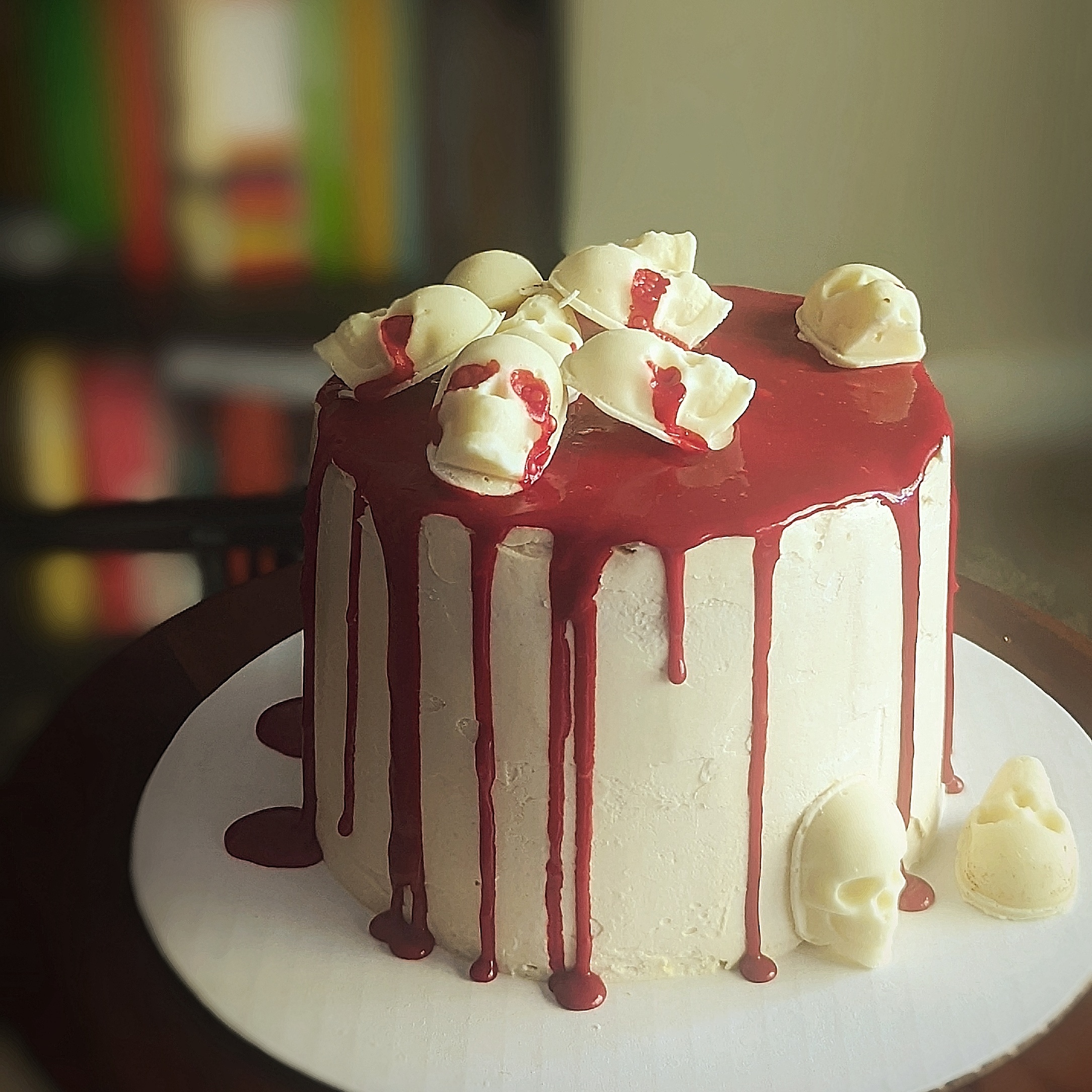
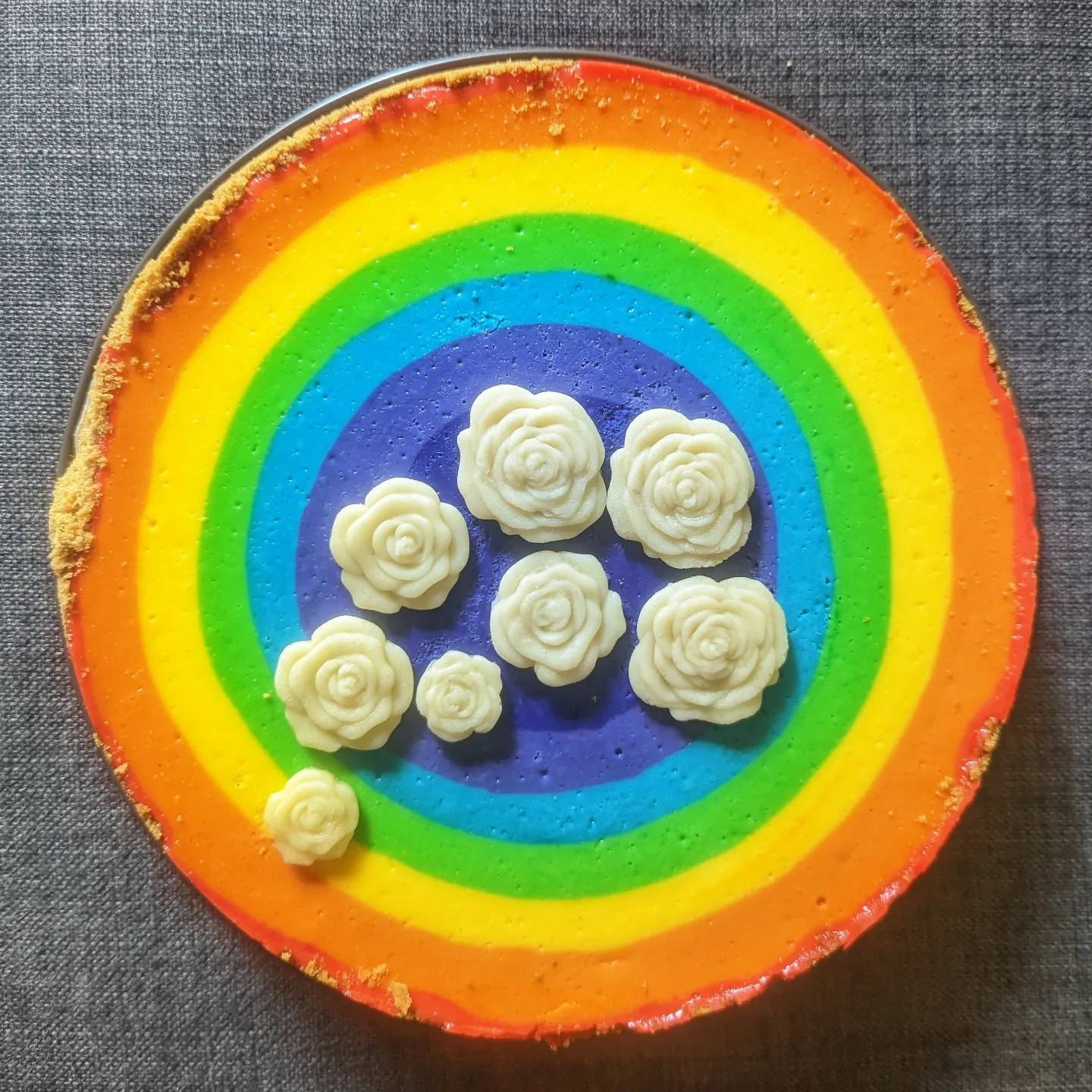
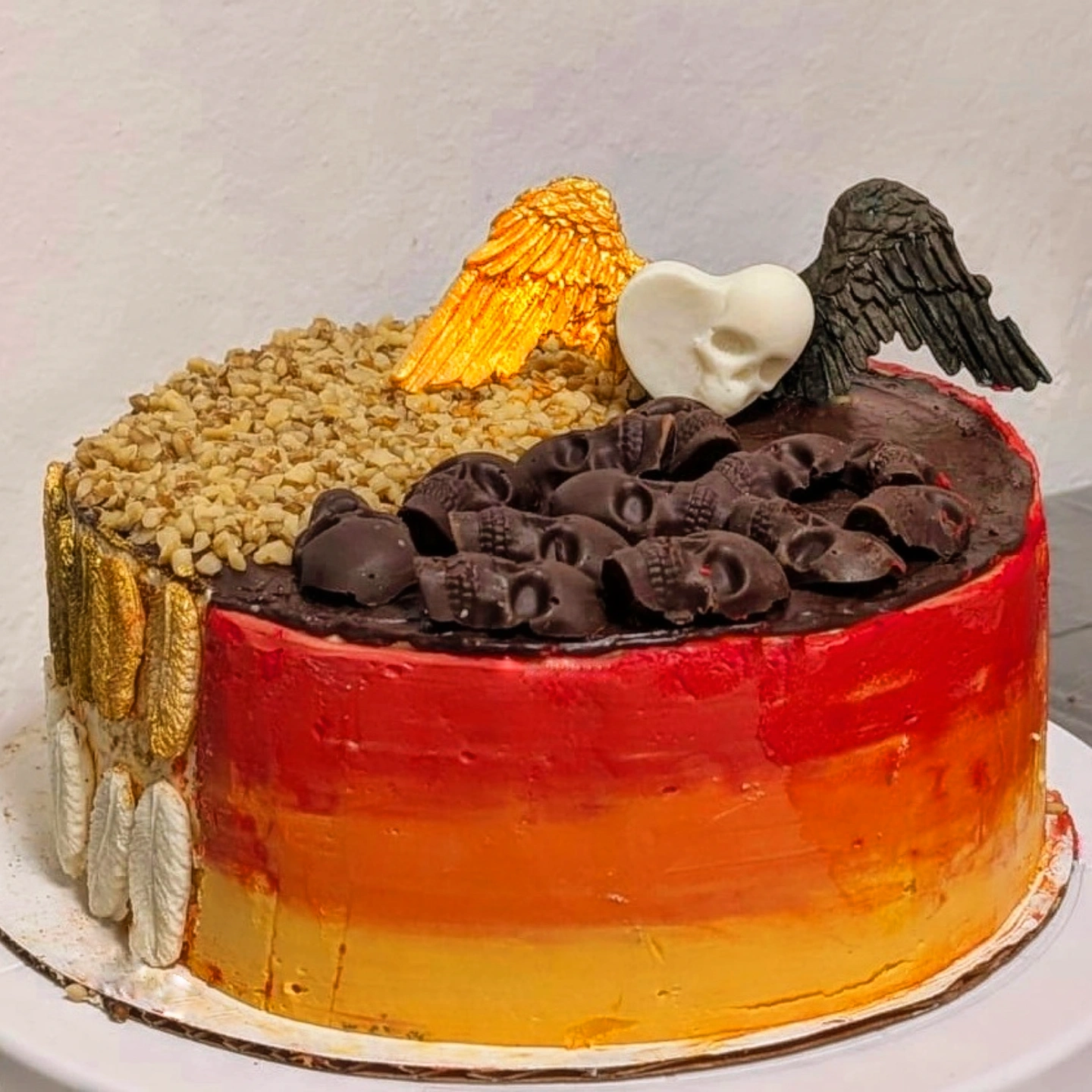
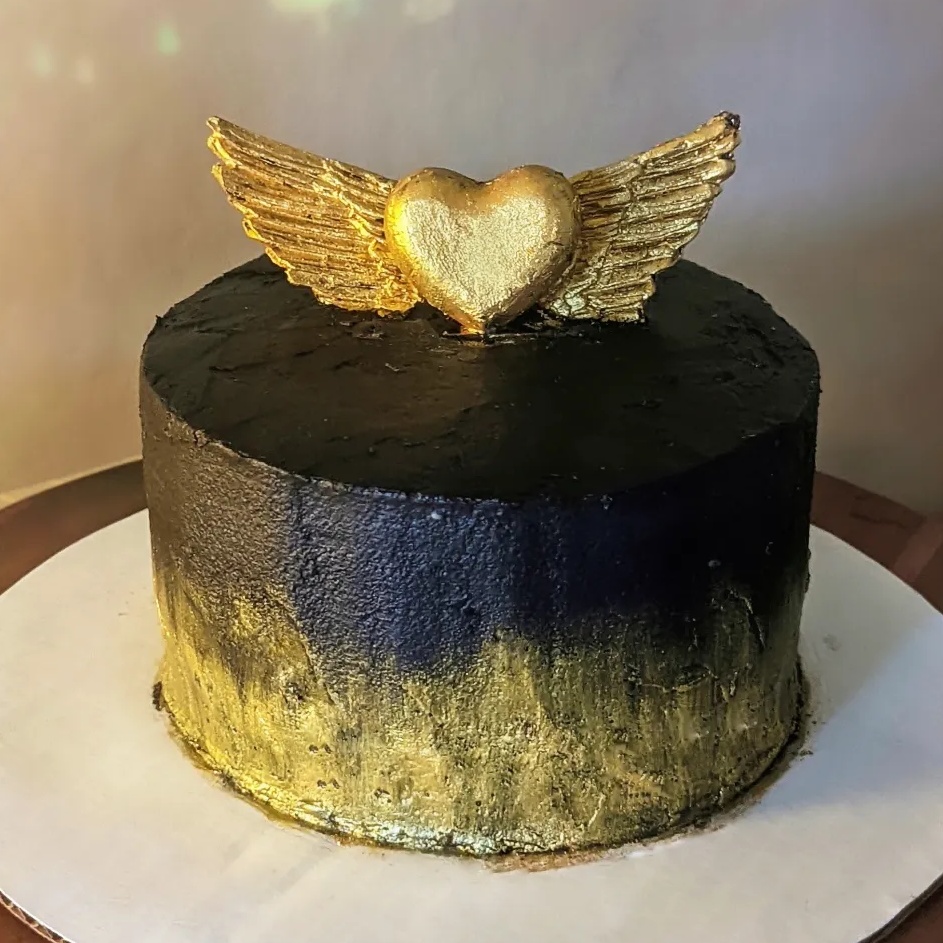
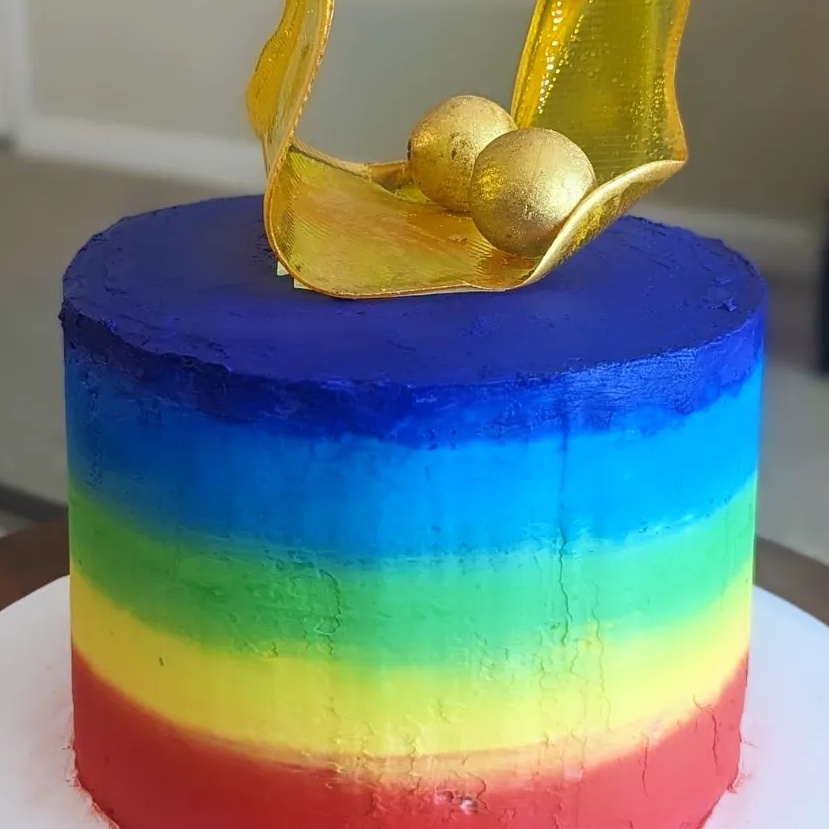
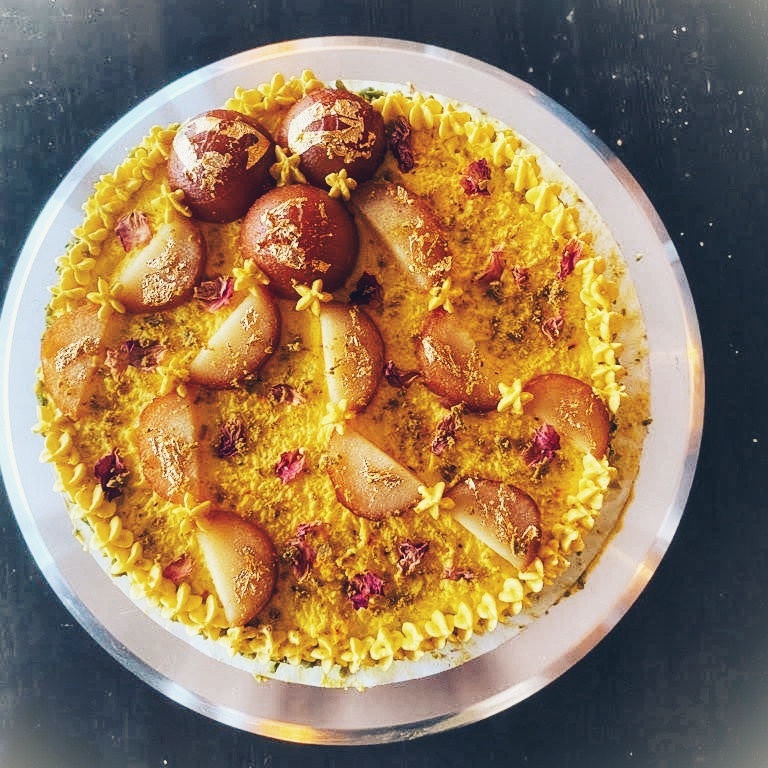
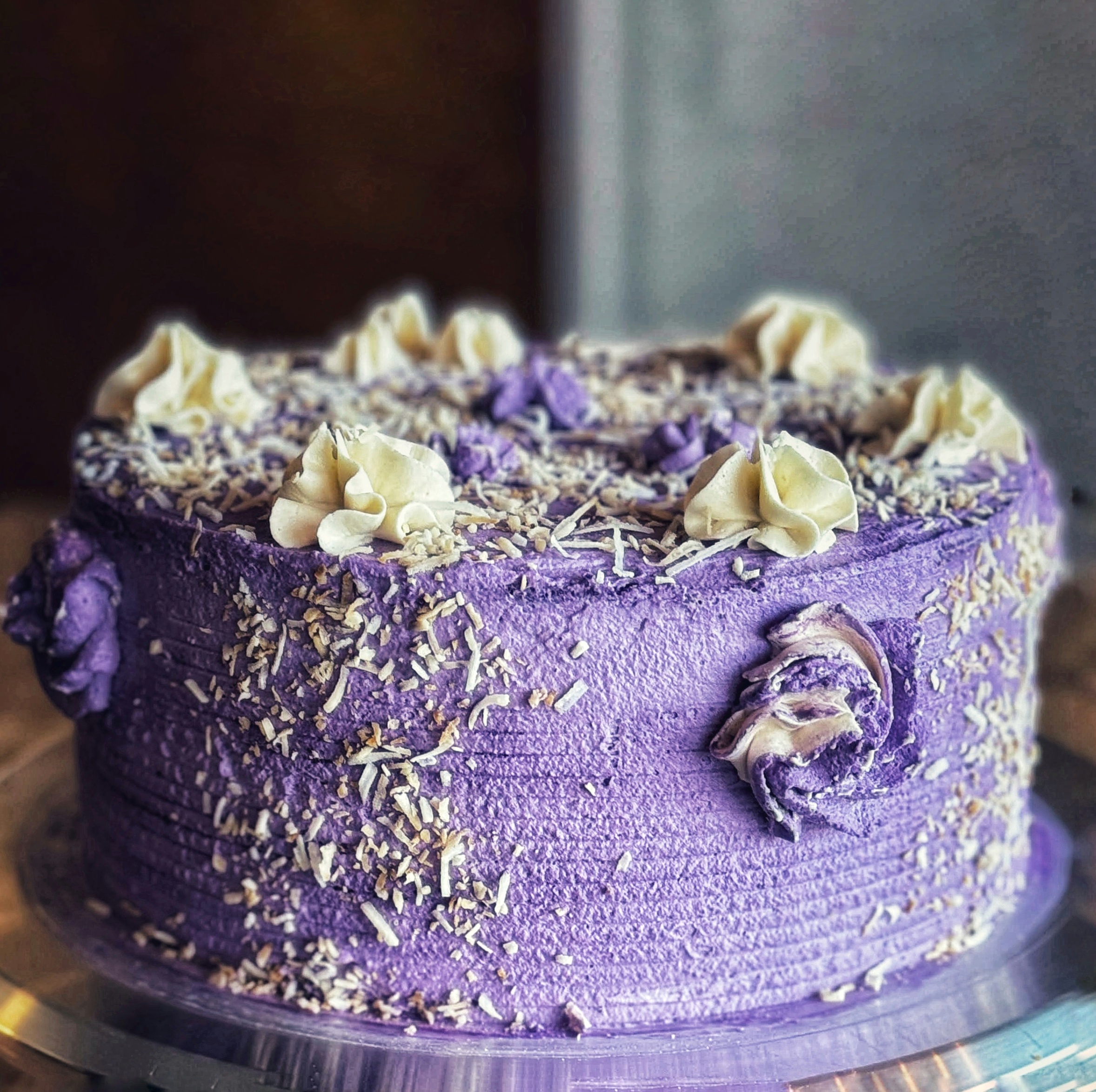
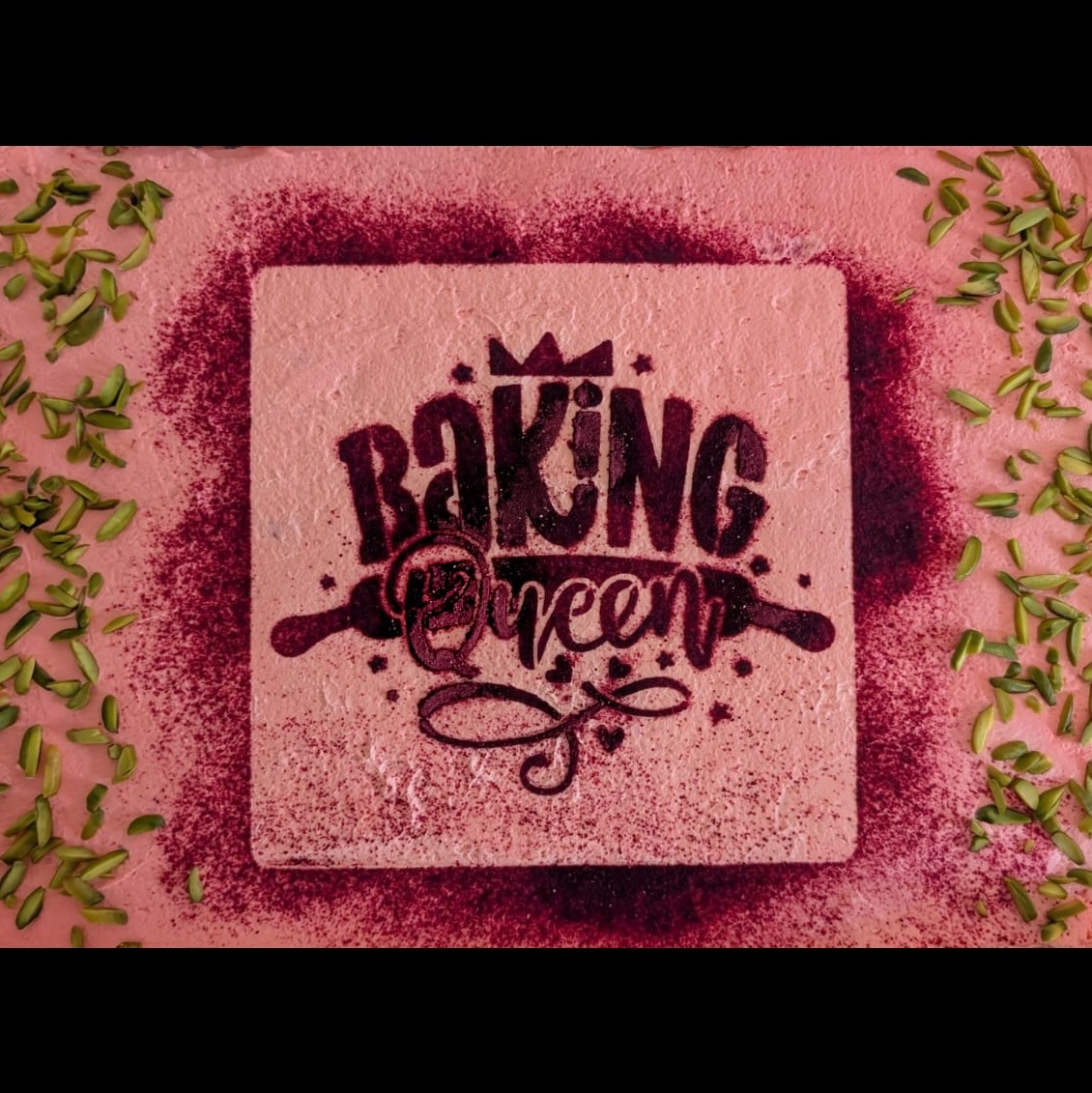
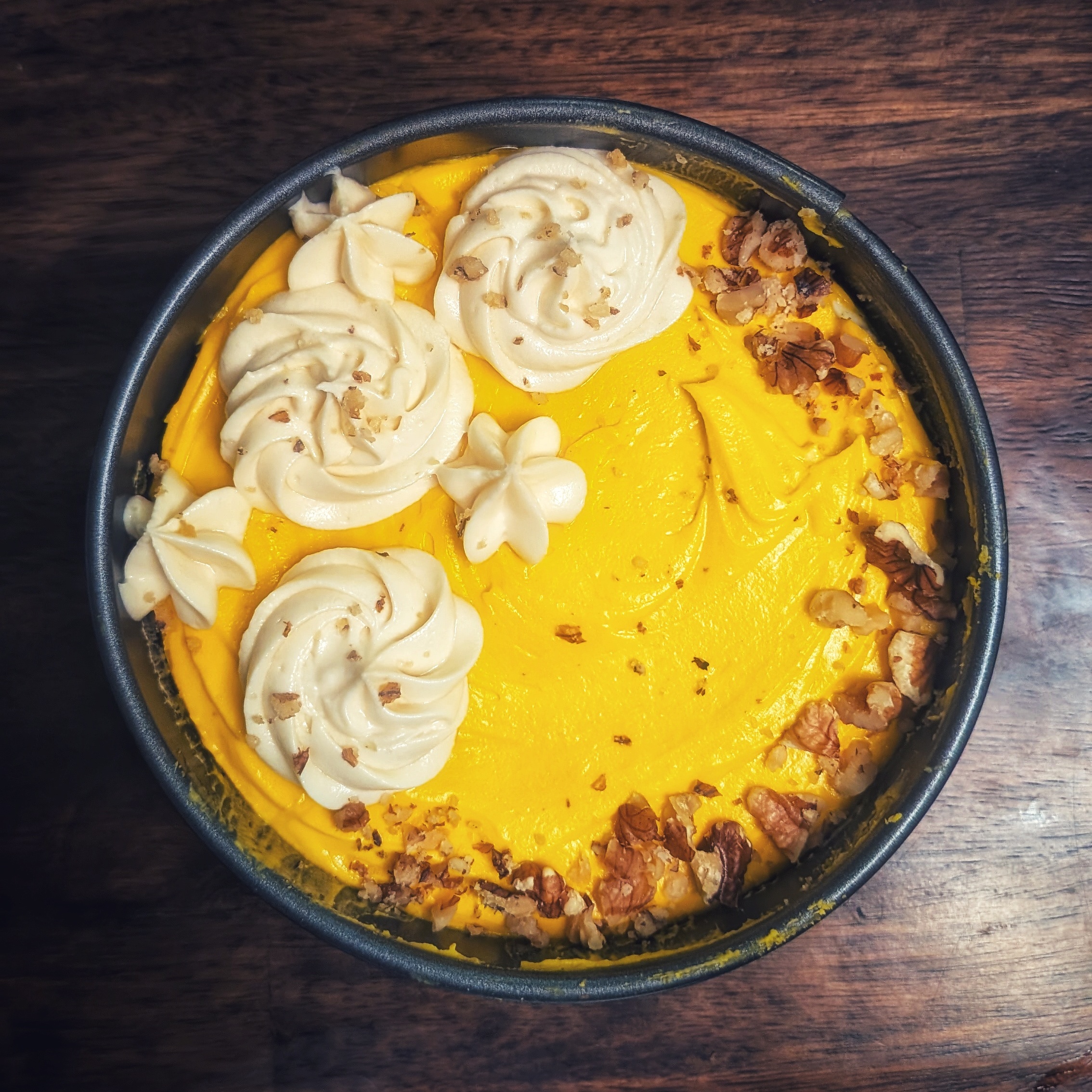
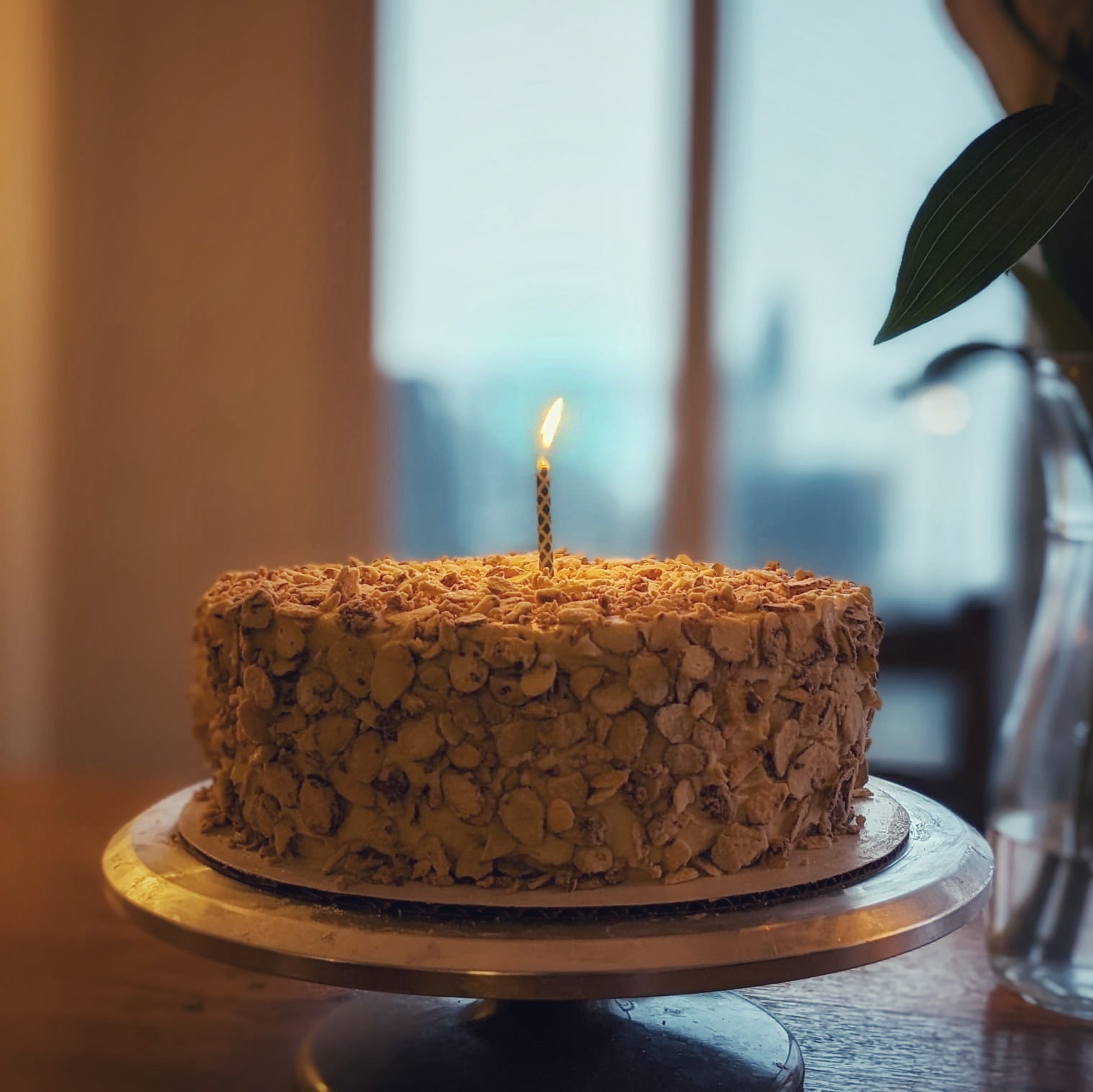
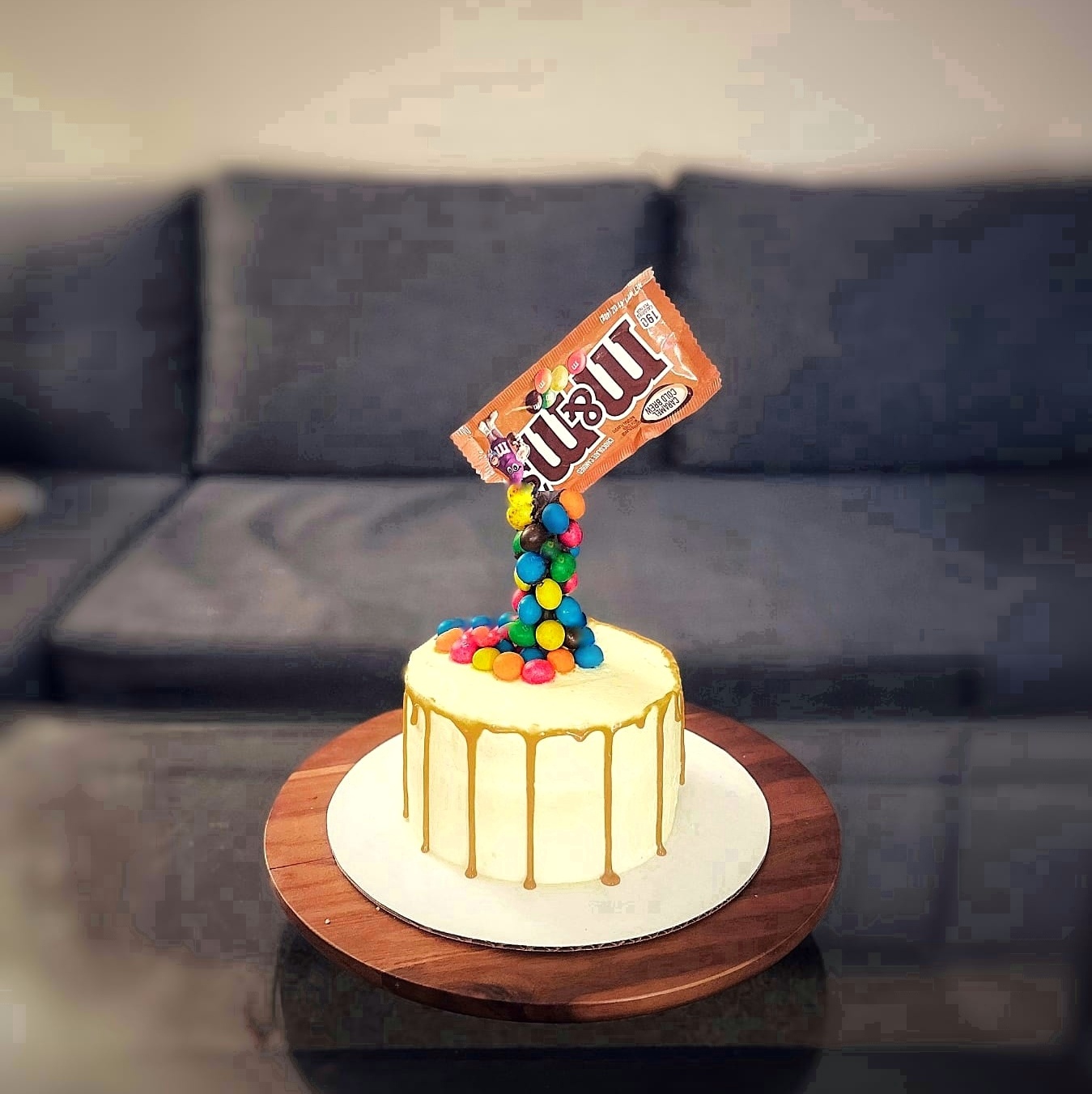
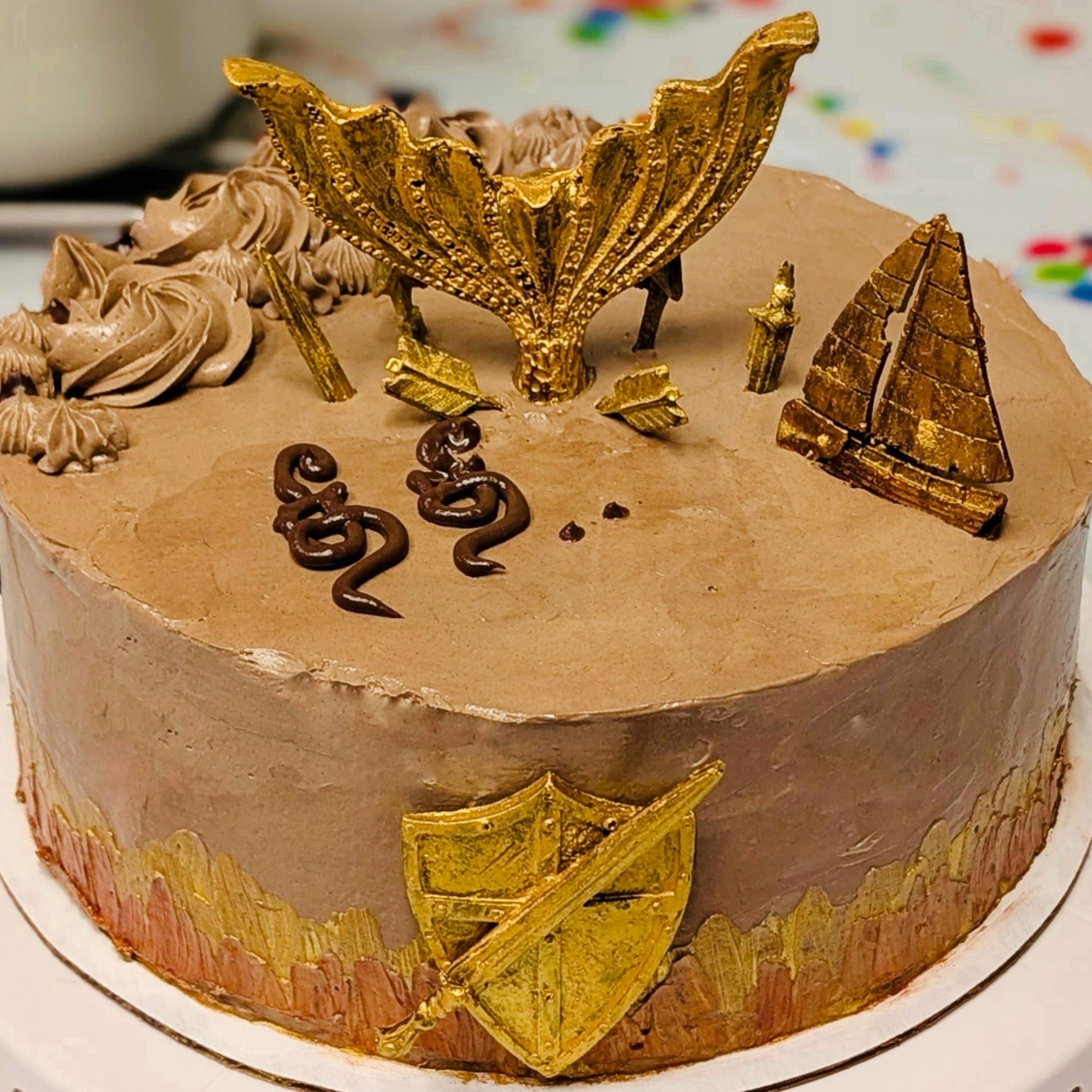
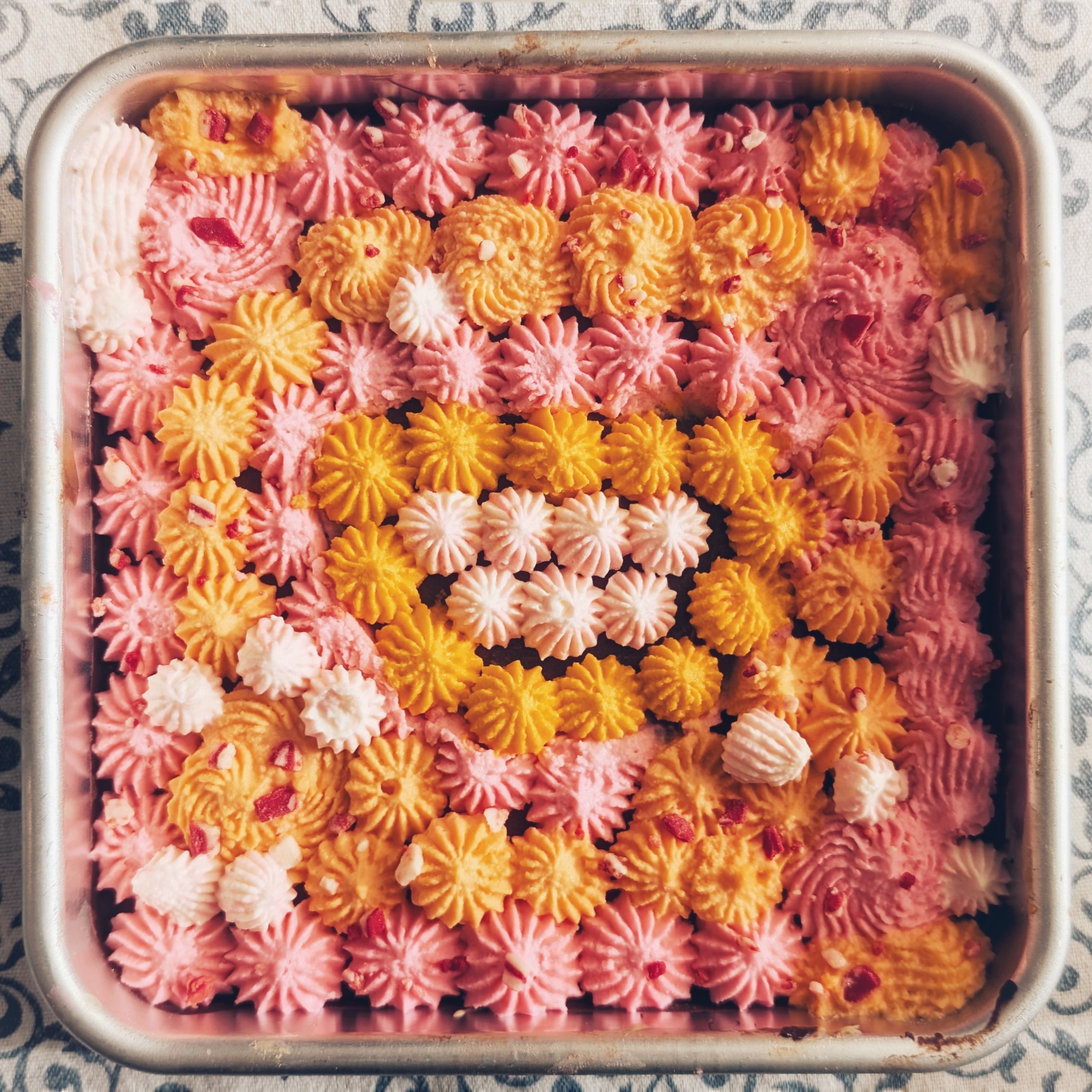
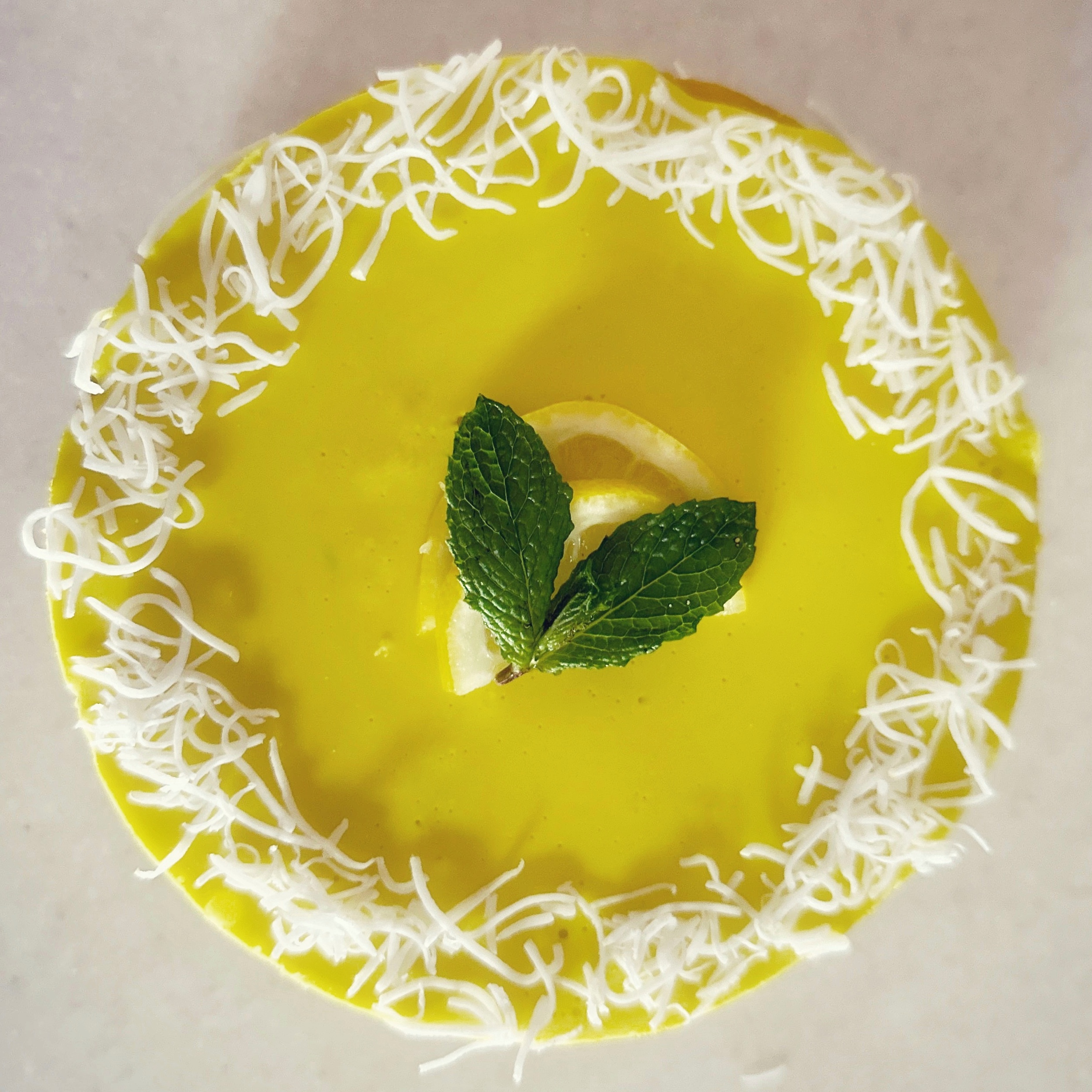
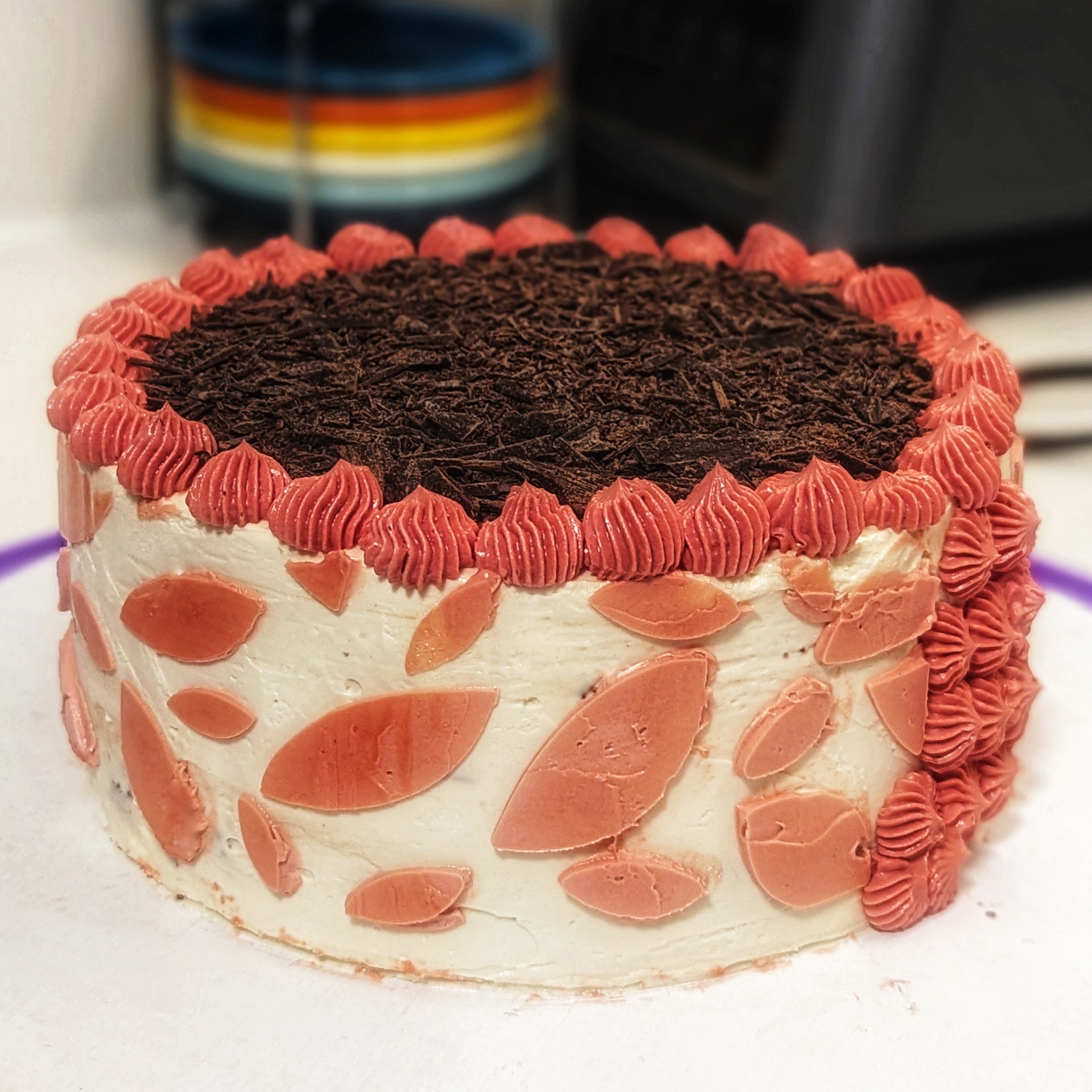
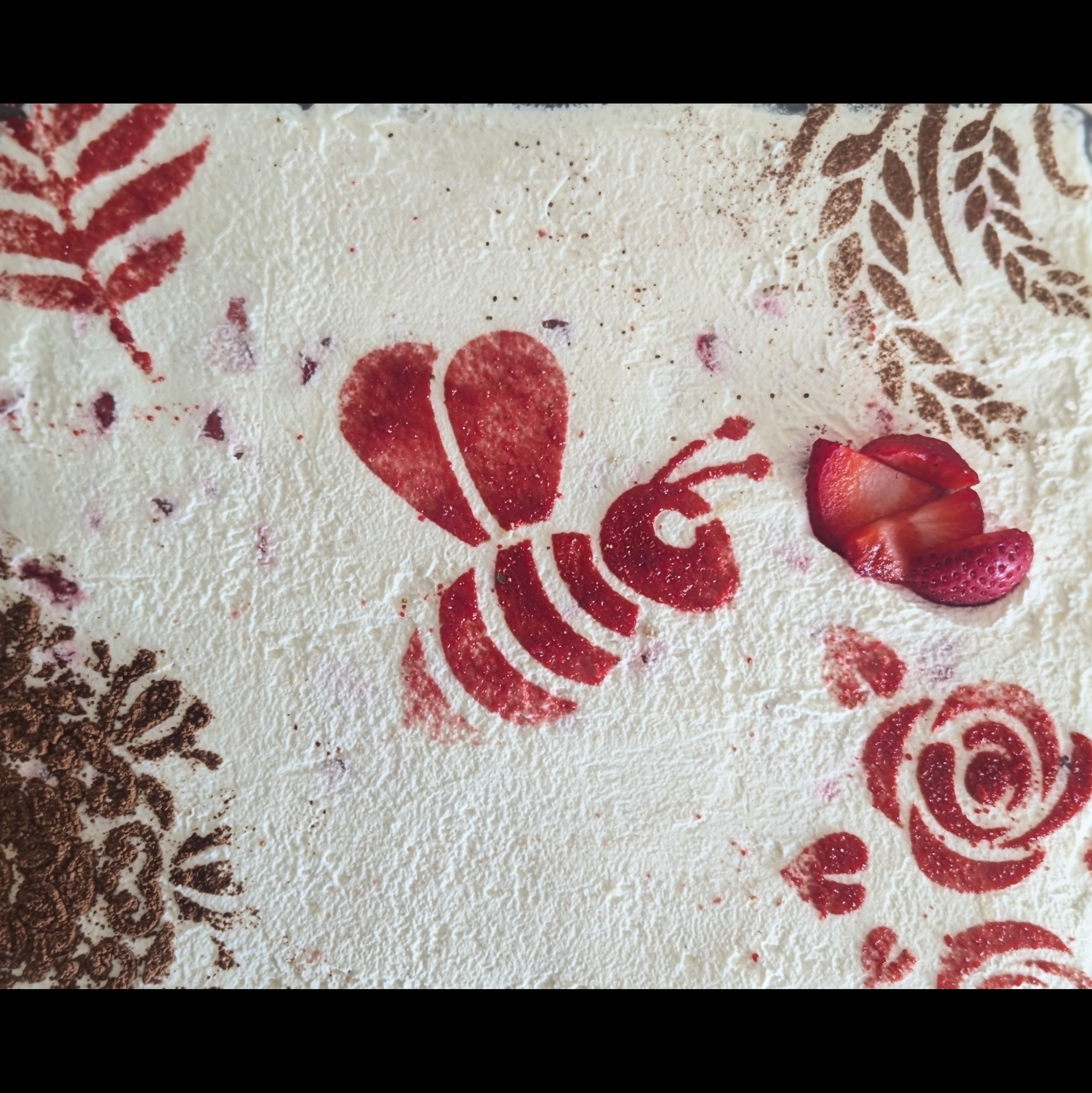
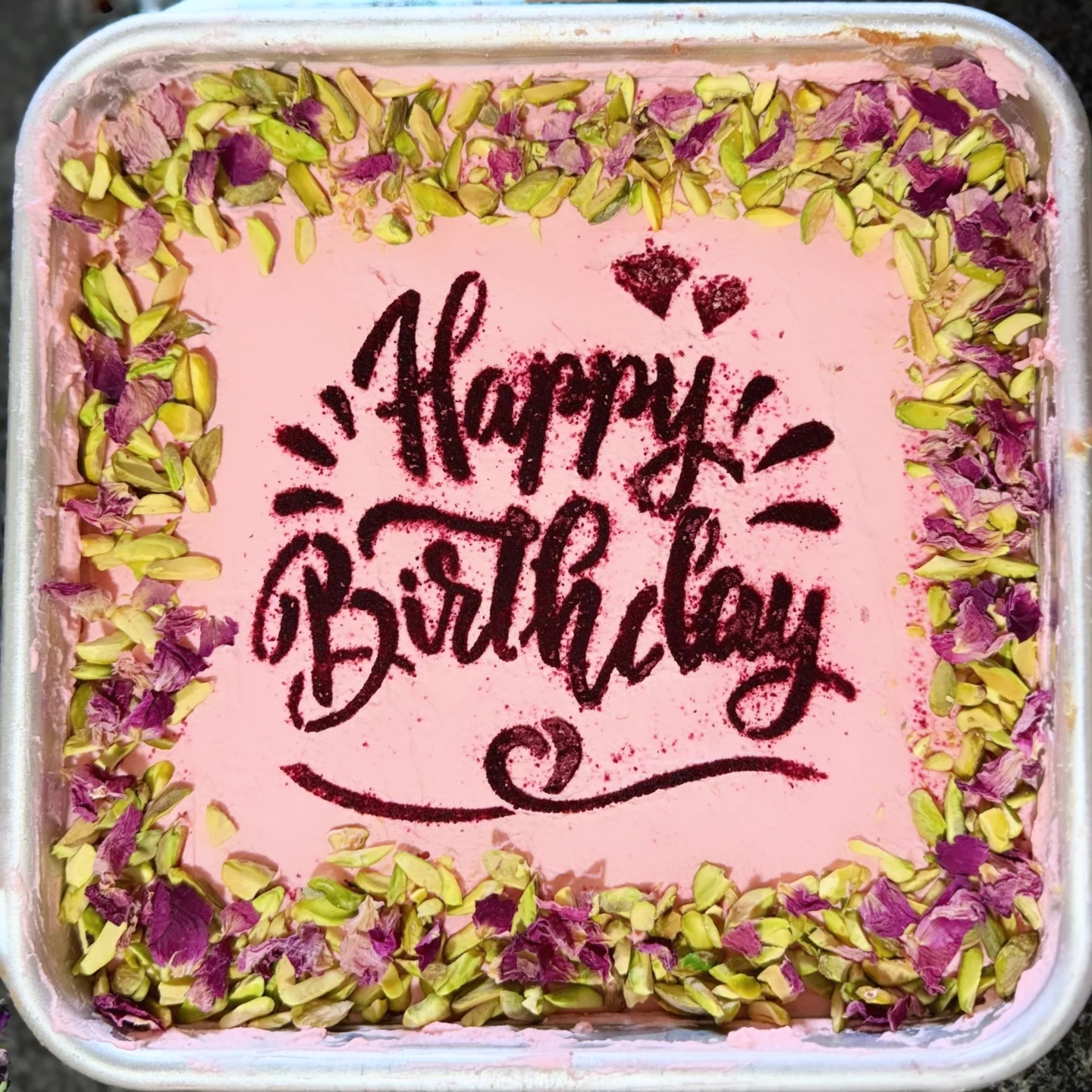
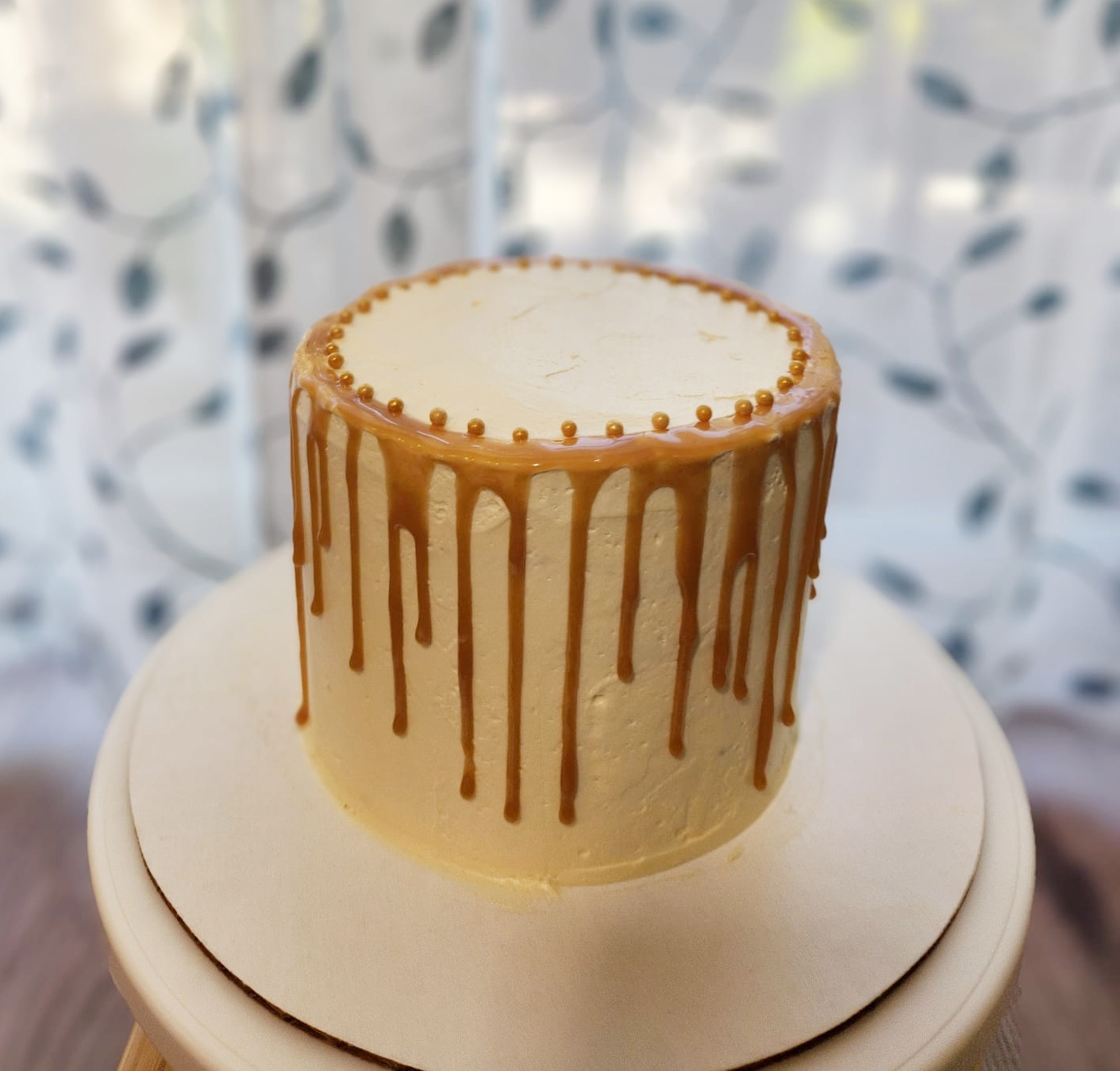
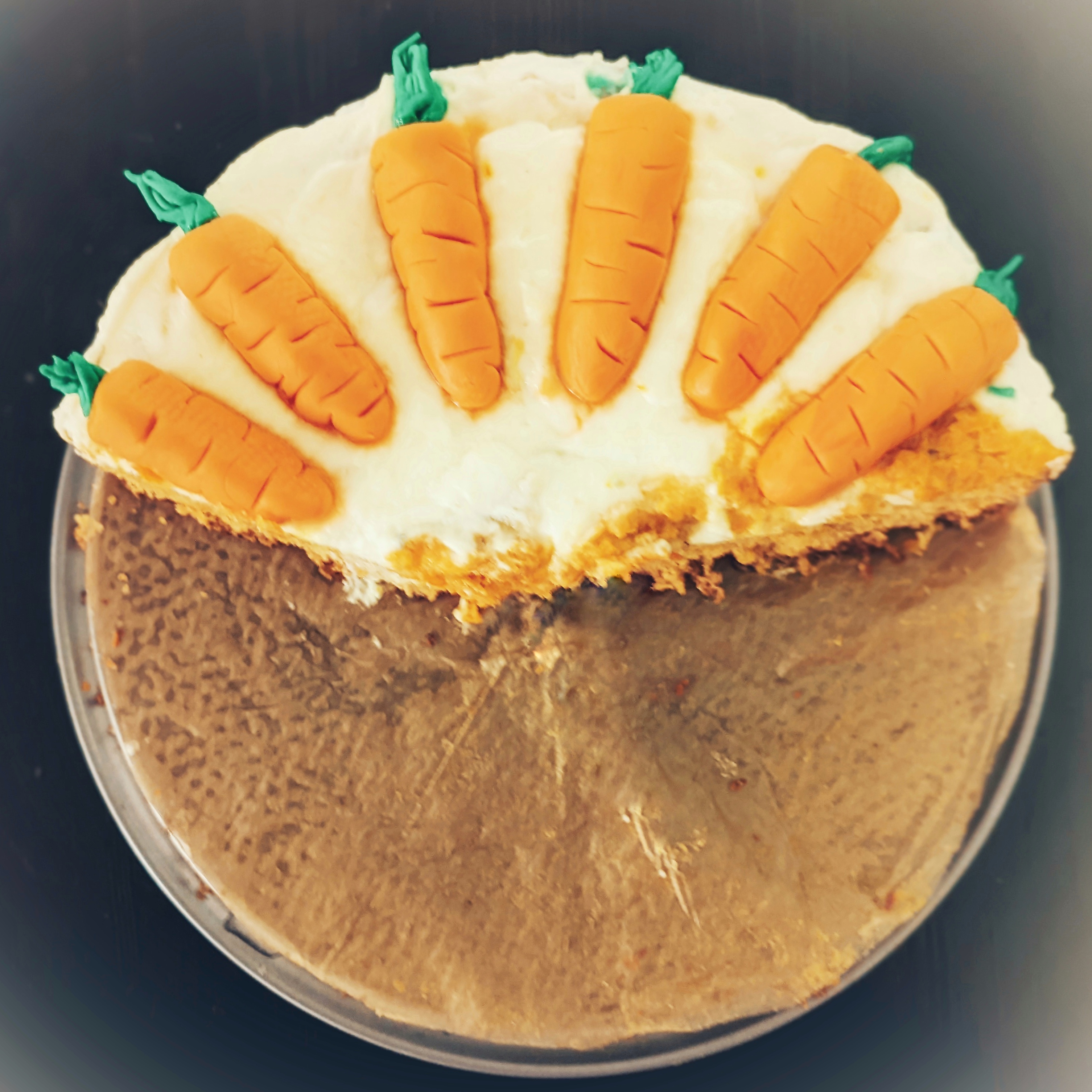
Required revisiting the classical problem of learning to abstain for modern settings (NeurIPS 2022, ICLR 2024b Spotlight, ICLR 2024c).